By Clare Flynn Levy
Share post
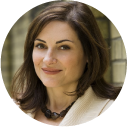
Clare Flynn Levy is CEO & founder of Essentia Analytics. Prior to setting up Essentia, she spent 10 years as a fund manager, in both active equity (running over $1bn of pension funds for Deutsche Asset Management), and hedge (as founder and CIO of Avocet Capital Management, a specialist tech fund manager).
The use of machine learning technology to identify patterns or signals in large data sets has a dizzying range of applications for businesses – from credit scoring and language processing, to facial recognition and online shopping nudges driven by insights into our past browsing behavior.
These businesses are essentially converting data into revenue. No surprise, then, that many investment managers are asking themselves how they can use machine learning to process the vast amounts of available market data into successful decisions on allocating capital.
This is the right question to ask, and portfolio managers ignore big-data analytics at their own peril. But there’s a hitch: financial and market data is much too complex and noisy for the “black box” machine learning that is transforming areas such as online advertising.
There’s a big difference between letting a black box dictate which ads you see on Facebook, and letting a black box inform your investment decisions. When you’re a fund manager, the financial risk of acting on noise rather than signal is massive.
To be successful with machine learning, professional investors must think outside the black box.
Backing the right horse
No one is more pleased than I am about the newfound appetite for innovation amongst asset managers.
During my decade as a portfolio manager, I was painfully aware how slow the sector could be to adopt new technologies and techniques for making investment decisions. So I’m thrilled to see data analytics and machine learning becoming a focal point in the asset management industry.
But as Marcos López de Prado, author of Advances in Financial Machine Learning and AQR’s Head of Machine Learning, noted recently in the Financial Times, professional investors need to appreciate the difference between the kind of ‘data-mining’ machine learning used by companies like Amazon, Google and Netflix, and the more theory-led version of machine learning that is appropriate for the noisy data sets that comprise the financial markets.
Black box machine learning will find patterns in your data and can provide recommendations based on what it has found. But it does so ‘blindly’ – ie it has no notion of cause and effect and does nothing to evolve the kind of concrete investment insights that allocators are looking for in a sustainable decision-making process.
Financial data is messy
Market data is very noisy, making clear signal detection difficult. It is subject to profound impacts from exogenous sources, outlying “fat-tail” events that are rare but drastic, and a host of biases implicit in the data itself (eg, survivorship bias).
It also displays a historical paucity, such that stock data from decades ago is less robust than current data – and certainly much less robust than, for example, the image data used to train Facebook’s facial recognition algorithms. (The rise of quant and passive investing has only exacerbated that issue: non-human decision-making is driving more and more of the decisions that appear in market data sets, whereas ten years ago, computer-led investment decisions were the minority).
Today, even the world’s most advanced quant funds will tell you that chucking black box machine learning at market data doesn’t result in a predictive model with any kind of resilience over time.
Left to their own devices, such machine learning techniques tend to “overfit” – overreaching in their quest to identify patterns and producing false positives or spurious correlations that any experienced investor would dismiss.
The way forward
These considerations don’t mean that machine learning is irrelevant in investing – far from it. It’s just a different type of machine learning that is required.
More suitable, in this context, is the “theory-based/scientific paradigm” approach. Here, machine learning is used to rapidly interrogate the data, testing out theories which are based on real-world investment experience.
At Essentia, machine learning helps us cut to the chase on understanding investment decision-making behavior. It is what has enabled us to analyse portfolio data and accurately identify meaningful behavioral patterns that lead to measurable performance improvement and the mitigation of behavioral bias.
In practical terms, we’re talking about the difference between a black box approach that tells you your best stock picks all have an “e” as their second letter, and a more nuanced analysis that can prove whether you have a tendency to over-trade during summer months, and if so, whether that behavior is specific to a certain sector, market cap, idea type, and so on.
One of those is far more likely to be a significant, sustained correlation, and therefore more relevant to any effort toward behavioral change and performance improvement. The other one is likely a red herring.
It goes without saying that knowing what theories to test is easiest if you’ve “sat in the seat” before – ie you truly understand the conditions in which the investor makes decisions. This real-world, organic experience “tames” the black box. Drawing investment conclusions from a massive raw data set and an unbridled algorithm is a high road to nothing, given the noise.
It was Plato who said ‘A good decision is based on knowledge and not on numbers’.
Machine learning is pushing the frontier of data exploration. But it can be a dangerous game – particularly in finance.
Investment managers seeking to leverage this powerful capability would do well to adopt a theory-based approach that is grounded in applied science and which factors in the innate, characteristically messy nature of the data available.
Hear Clare Flynn Levy discussing why active management is ripe for AI and how it can help human fund managers play to their strengths. Listen >
Related reading:
Asset Managers Plan to Boost AI Spending, a Greenwich Survey Shows (Institutional Investor)
Overfitting in Machine Learning – An Explainer (Elite Data Science)
The Big Problem With Machine Learning Algorithms (Bloomberg)
Hedge funds embrace machine learning – up to a point (The Economist)